Simplified access to QML methods
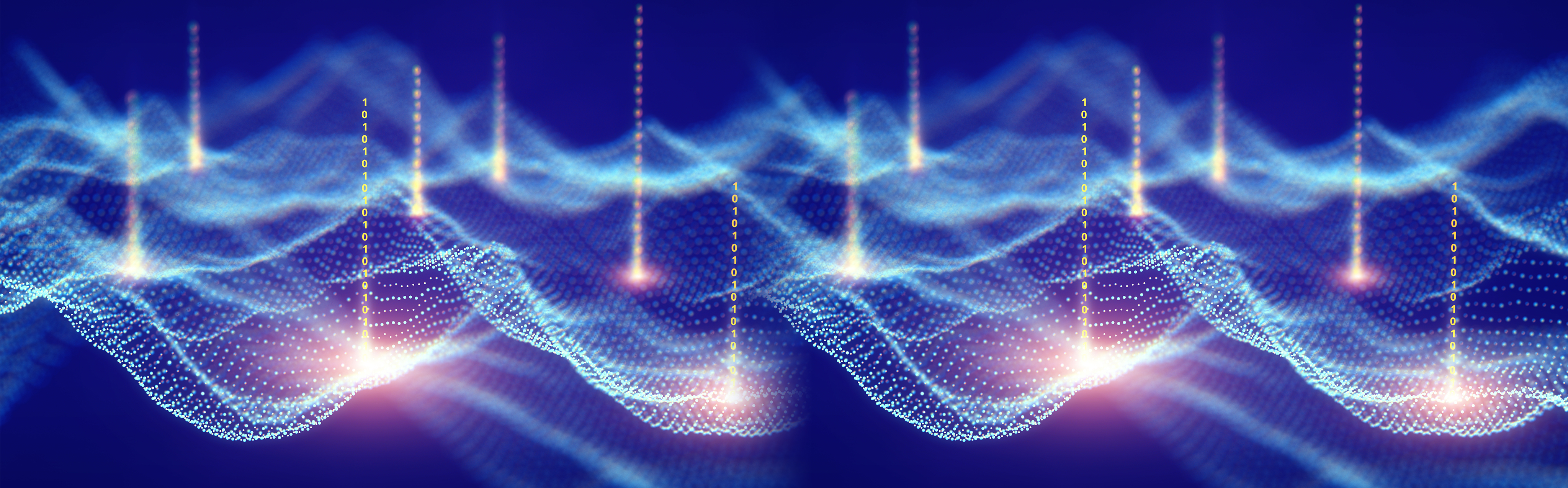
The implementation of machine learning solutions is still associated with a high workload for companies. From data acquisition, to the selection of suitable algorithms, to the optimization of training, detailed expertise in the respective application domain is necessary. The approach of automated machine learning (AutoML) can help to simplify the use of ML processes. The AutoQML project supports developers without deep ML expertise to solve typical problems with dynamic ML methods. This is a forward-looking way to address the skills shortage in this environment. However, this gain in user-friendliness is at the same time offset by very high hardware requirements, which go hand in hand either with significant investments of one's own or with outsourced cloud computing and a corresponding loss of one's own data sovereignty. Similar developments can be expected in quantum computing. In Quantum Machine Learning (QML), quantum computing promises to solve certain problems much faster than conventional computing power. However, a core challenge here also remains the shortage of skilled workers. To enable broad, industrial application of QML, a high degree of user-friendliness and automation is essential.
The AutoQML project therefore aims to both solve the challenges of current AutoML approaches and to adapt the successful approach of AutoML. Future users should thus have easier access to QML methods.
Based on use cases from the automotive and production area, new tools, components, methods and algorithms for machine learning with quantum computers will be developed. The tools and methods developed in the project will be integrated into the PlanQK platform as an open source solution and thus made available to (quantum) developers. In addition, new quantum computing software for automated machine learning will be developed, in particular for hybrid QML algorithms and hyperparameter optimization using quantum computing.
The AutoQML project aims to provide conventional developers with easy access to both classical and quantum ML algorithms including hyperparameter optimization. In doing so, (Auto)ML experts will be provided with quantum solutions in order to be able to design hybrid overall solutions that make use of the best components from both worlds, depending on the application. Key results are: